Numerous technologies are being implemented in orchards with the aim of improving orchard management. Among them, orchard yield mapping is being used to optimise production and resource management. The integration of the Global Navigation Satellite System (GNSS) and other sensors has been made possible by advances in microcomputers, which have enabled complex algorithms to be implemented in the field at low cost.
The You Only Look Once (YOLO) algorithm is the most popular one-step object detection model. The advantage of YOLO is the faster inference time compared to two-stage detectors, which allows it to be used in real-time scenarios. DeepSORT (simple online real-time tracking with deep association metric) is a multi-object tracking approach that shows outstanding performance.
Increasingly, these technologies are being implemented in orchards, but little attention has been paid to this approach in sour cherry management. The objective of the investigation conducted at Utah State University in Logan, USA, was therefore to evaluate two methodologies for monitoring sour cherry yield: one that incorporates proximity sensors for detecting changes in the harvest tank and the other that employs computer vision for real-time fruit counting.
The objectives of the first approach consist of analysing harvest statistics and yield maps, while the objectives of the second approach include evaluating the inference rate and accuracy of fruit counting. The initial approach employs proximity sensors, Raspberry Pi and GPS to estimate yield variability by monitoring tank variations during harvest.
The second method uses computer vision, specifically YOLOv8 and DeepSORT, to determine the quantity of cherries per tree. During the harvest phase, the operators maintained a constant rate of tank loading, with an average of 516 kg per tank. The average block yield was 9.16 t/ha. The yield map illustrated the spatial variations, and the data obtained from the instrument offered valuable insights into harvest efficiency.
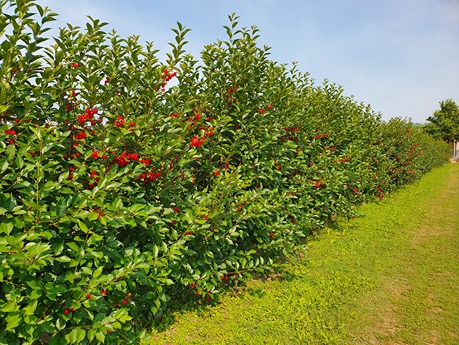
The different training times of the tested models clearly illustrates the trade-off between accuracy and speed, while underlining the suitability of YOLOv8n for real-time fruit counting. These methods, which employ hand-held computers, offer substantial improvements in yield mapping for precision agriculture, thus providing valuable insights for fruit growers.
The proposed methods show considerable potential as effective yield monitoring tools. In the orchard under investigation, the measurements indicate that the variation between operators in terms of the filling of harvest tank is minimal. In the future, the model will be trained using scenarios containing different levels of occlusion, movement distortion and lighting variations.
It will be possible to understand the influence of adverse conditions on the accuracy of the model by calculating metrics in these different scenarios. Further investigations are needed to realise the full potential of these findings, which have significant implications for the development of precision agriculture in cherry orchards.
Source: Safre, A., Torres-Rua, A., Black, B. and Schaffer, B. (2024). Advanced methods for yield mapping in tart cherries: tank change tracking and YOLO-DeepSort fruit counting. Acta Hortic. 1395, 289-296, https://doi.org/10.17660/ActaHortic.2024.1395.38.
Image: SL Fruit Service
Melissa Venturi
University of Bologna (IT)
Cherry Times - All rights reserved